Table of Contents
Introduction to Replicate AI
The field of artificial intelligence (AI) has seen rapid advances in recent years, with the development of powerful new techniques like deep learning and transfer learning. One emerging approach that holds great promise is Replicate AI – the concept of replicating, reproducing, or “cloning” existing AI models.
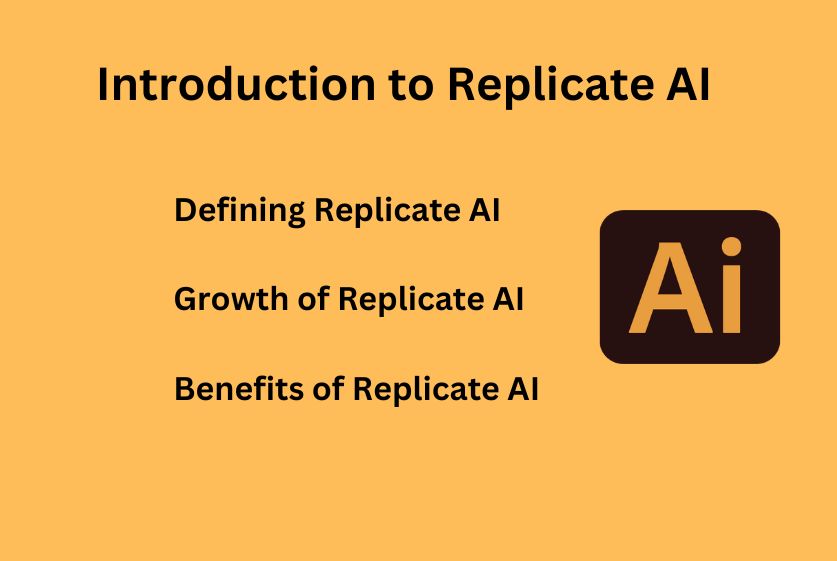
Defining Replicate AI
Replicate AI refers to the process of building AI systems that aim to mimic the functionality of existing models developed by other organizations. The goal is to reproduce the key behaviors and outputs of mature, proven models by studying them and training similar models on the same types of data.
For example, a tech company may analyze the famous GPT-3 model created by OpenAI and then attempt to develop its own natural language model that functions in highly similar ways, albeit on a smaller scale. The core techniques would be replicated as much as possible.
Growth of Replicate AI
Interest and investment in Replicate AI have accelerated recently for several reasons:
- Rapid progress in deep learning and related areas provides a foundation for reproducing state-of-the-art models.
- Many advanced models are proprietary and inaccessible, motivating parallel efforts.
- Replicating successful models is more feasible than designing novel architectures.
- Licensing models from vendors can be prohibitively expensive.
Replicate AI enables more organizations to gain the advantages of powerful AI without massive overhead costs.
Benefits of Replicate AI
Done ethically and legally, pursuing Replicate AI can offer major benefits:
- Reduced costs – Replicating models is more affordable than licensing them.
- Customization – Models can be tailored to specific use cases.
- Interoperability – Technical integration is simplified when models share architectures.
- Responsible innovation – Organizations can ensure models align with their values.
The potential to democratize access to advanced AI warrants further exploration.
Key Players in Replicate AI Landscape
Many tech firms are now participating in the Replicate AI ecosystem in some capacity. Some of the major players include:
Anthropic
Founded by former OpenAI researchers, Anthropic focuses on developing safe and beneficial AI systems. Their Claude model replicates capabilities like constitutional reasoning.
Cohere
Cohere provides NLP models-as-a-service like Cohere API. They aim to reproduce the strengths of models like GPT-3 while optimizing for precision and accuracy.
Stability AI
Stability AI is the startup behind the image generator Stable Diffusion. They are replicating and iterating on advanced diffusion models for generative art.
These companies and others demonstrate the early potential of Replicate AI across areas like generative content, reasoning, and chatbots.
Replicate AI Model Architectures
There are two major machine learning architectures that current Replicate AI efforts target: transformer models for sequence tasks and diffusion models for image generation.
Transformer Models
Transformers utilize attention mechanisms to analyze relationships between all words in input sentences. Their ability to handle long-range dependencies makes them well-suited for language tasks.
GPT Models
Models in the GPT (Generative Pretrained Transformer) family pioneered by OpenAI are among the most replicated, thanks to breakthrough natural language performance.
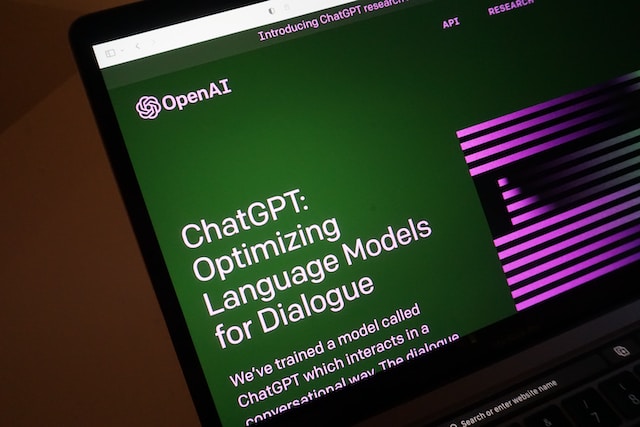
Codex Models
Codex models like GitHub’s Copilot are also being reproduced. They generate computer code in various programming languages, accelerating software development.
Diffusion Models
Diffusion models create images pixel-by-pixel based on noise reduction across sequential densified steps.
DALL-E Models
OpenAI’s DALL-E architecture serves as inspiration for many replicated generative art models.
Stable Diffusion
Models based on Stability AI’s Stable Diffusion are among the most prominent and allow high-fidelity image generation using text prompts. In both categories, established models provide proven templates for replication.
Training Data for Replicate AI Models
While model architecture is essential, training data plays an equally vital role in determining replicate model capabilities. There are some key considerations around curating data for Replicate AI systems:
Quality Data Curation
Training data must be sufficiently voluminous and cover a diverse range of high-quality examples for the target domain. Low-quality or restricted data will limit performance.
Removing Dataset Bias
As replicate models may inherit and amplify biases, it is critical to proactively analyze and address problematic elements in the training data.
Expanding to New Domains
Finding creative ways to produce training examples for new niche areas will help replicated models generalize better. Synthetic data generation may be valuable.
For instance, an art model would need a vast dataset spanning different styles, mediums, genres, and techniques to properly inform replication.
In data lies the difference between mediocrity and excellence for Replicate AI.
Replicate AI Applications
Replicate AI powers use cases across many industries and domains, from creative fields to business operations.
Creative Applications
Many replicated transformer and diffusion models excel at generative applications for the arts and entertainment sectors.
Generative Art
Text-to-image models like DALL-E clones create original digital images, illustrations, or photos based on text prompt inputs.
Interactive Fiction
Some language models replicate capabilities seen in tools like AI Dungeon for text adventure games and interactive fiction.
Business Applications
Meanwhile, enterprises leverage Replicate AI for more applied scenarios through services provided by vendors.
Customer Service Chatbots
Replicated transformer architectures field customer queries and conduct conversational interactions.
Content Creation
Marketing content, reports, and other business writing can be automatically composed by replicate language models. These use cases highlight the versatility of reproduced models.
Ethical Considerations for Replicate AI
As with any rapidly evolving technology, there are ethics and responsible innovation concerns to consider regarding the burgeoning Replicate AI landscape:
Potential for Misuse
If deployed without sufficient safeguards and monitoring, replicated generative models could enable various harms.
Deep fakes
Realistic media forgery using AI, known as deep fakes, could impersonate public figures or non-consenting individuals for malicious purposes.
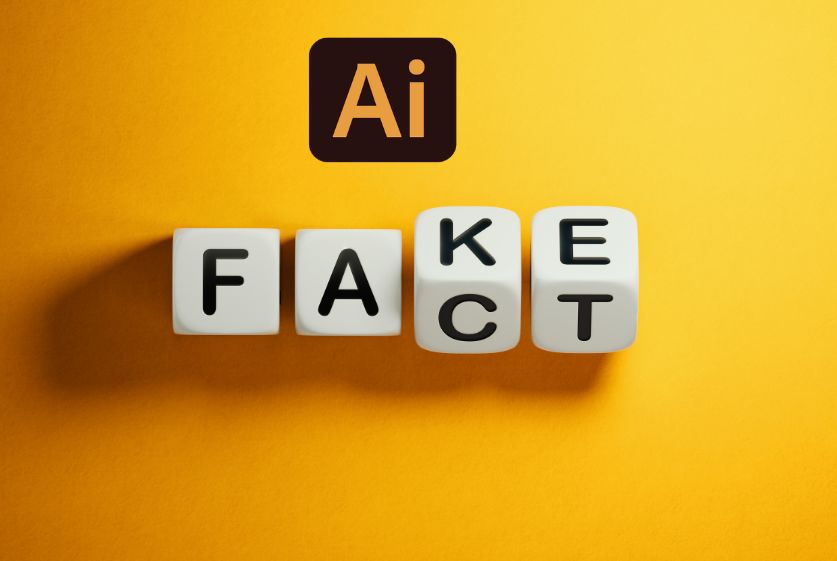
Misinformation
Automatically generated fake content at scale poses risks of spreading misinformation with societal impacts.
Lack of Transparency
Many details around training data and model decision processes for proprietary replicate models remain undisclosed.
Provenance Tracking
Mechanisms for tracking media provenance and attribution are needed for accountability.
Model Cards
Clear documentation of model strengths, weaknesses, and ethical screening processes in “model cards” helps ensure transparency. Careful foresight and governance of Replicate AI systems are paramount.
The Future of Replicate AI
Looking ahead, we can expect to see continued evolution in the sphere of Replicate AI:
New Model Architectures
Fresh architectures offer new frontiers for replica models, like multimodal models combining vision and language.
Specialized Models
Targeted replication will produce more domain-specific models tailored for niche applications.
Greater Accessibility
Simplified model interfaces and falling costs may finally enable the democratization of advanced AI capabilities to the masses.
If cultivated conscientiously, this new paradigm in AI development may profoundly transform how artificial intelligence impacts our world – perhaps in ways we cannot yet even imagine.
Conclusion
The emergent field of Replicate AI presents intriguing opportunities as well as risks. Reproducing powerful AI models promises expanded access and customization while necessitating added ethical diligence. Much remains to be discovered regarding where this technology path may lead us.
But by upholding principles and partnering thoughtfully with machines, our artificial intelligence journey lies open to creative progress for the common good.
FAQs
What are the main goals of Replicate AI?
The main goals are reproducing the key capabilities of advanced proprietary models via architecture patterns reuse, providing cheaper licensing alternatives, and customizing systems for specific use cases.
What are the potential benefits of the Replicate AI approach?
Benefits may include reduced costs, improved accessibility, the ability to tweak models according to specific needs, and responsible innovation control regarding ethics.
What are some examples of Replicate AI model architectures?
Prominent examples include transformer architectures like GPT-3 for language and Codex for code generation alongside diffusion models like DALL-E 2 and Stable Diffusion for generative image creation.
How is training data crucial for Replicate AI systems?
Sufficient data coverage, diversity, depth, and quality are vital to match the performance of the systems being replicated. Removing problematic biases also improves generalization.
What are some responsible innovation considerations around Replicate AI?
Key considerations include mitigating potential misuse cases, improving transparency and provenance tracking, establishing model documentation standards, and developing effective governance frameworks.