Table of Contents
What is Robust Artificial Intelligence?
In the rapidly evolving field of artificial intelligence (AI), the concept of Robust Artificial Intelligence has emerged as a critical area of focus. Robust AI refers to intelligent systems that are resilient, adaptable, and trustworthy, even in the face of uncertainties, adversarial attacks, or changing environments. These systems are designed to maintain high performance and reliability under a wide range of conditions, ensuring their practical applicability in real-world scenarios.
Importance and Applications
The development of robust AI is crucial for a multitude of applications, ranging from healthcare and cybersecurity to autonomous vehicles and financial systems. As AI systems become more integrated into critical infrastructure and decision-making processes, ensuring their robustness is paramount to minimize risks and maximize benefits.
Robust AI systems have the potential to transform industries, enhance efficiency, and improve decision-making processes, ultimately contributing to a safer and more reliable technological landscape.
Key Characteristics of Robust Artificial Intelligence Systems
Adaptability and Flexibility
One of the hallmarks of robust AI systems is their ability to adapt to changing conditions and learn from new data.
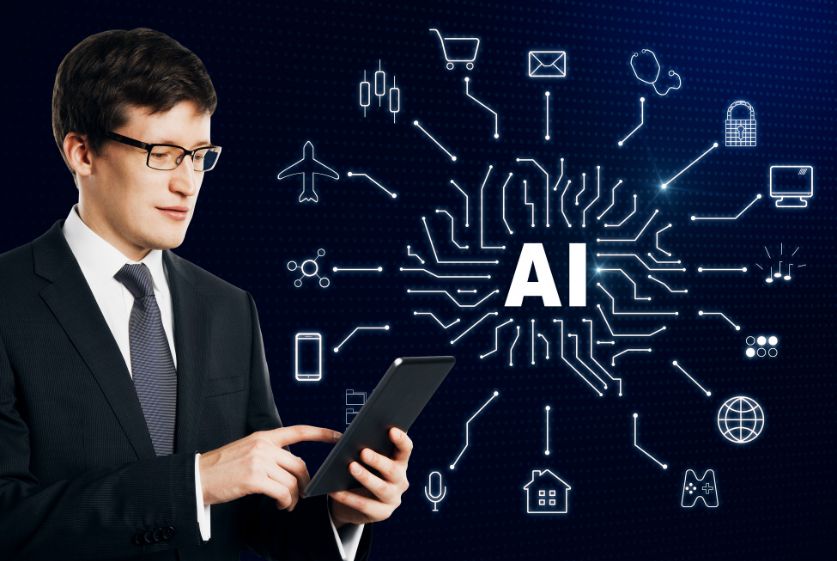
These systems are designed to continuously update their knowledge and refine their models, ensuring that they remain relevant and effective even as the environment evolves. This adaptability is essential for tackling complex, dynamic problems that require real-time adjustments and decision-making.
Reliability and Fault Tolerance
Robust AI systems must be reliable and fault-tolerant, capable of maintaining performance even in the presence of errors, noise, or adversarial attacks. These systems incorporate mechanisms to detect and mitigate potential failures, ensuring continuous operation and minimizing downtime. Fault tolerance is particularly crucial in applications where safety and security are paramount, such as autonomous vehicles or critical infrastructure monitoring.
Scalability and Efficiency
As AI systems become more prevalent and handle larger volumes of data, scalability, and efficiency are essential characteristics of robust AI. These systems must be able to scale up or down seamlessly, adapting to changing computational demands while optimizing resource utilization. Efficient algorithms and architectures are necessary to process data in a timely and cost-effective manner, enabling real-time decision-making and analysis.
Challenges in Developing Robust Artificial Intelligence
Data Quality and Availability
One of the primary challenges in developing robust AI systems is ensuring high-quality and diverse data. AI models are heavily reliant on the quality and representativeness of the training data, as biases or gaps in the data can lead to inaccurate or unreliable predictions. Obtaining large, diverse, and well-curated datasets is often a costly and time-consuming process, especially in specialized domains or when dealing with rare or extreme scenarios.
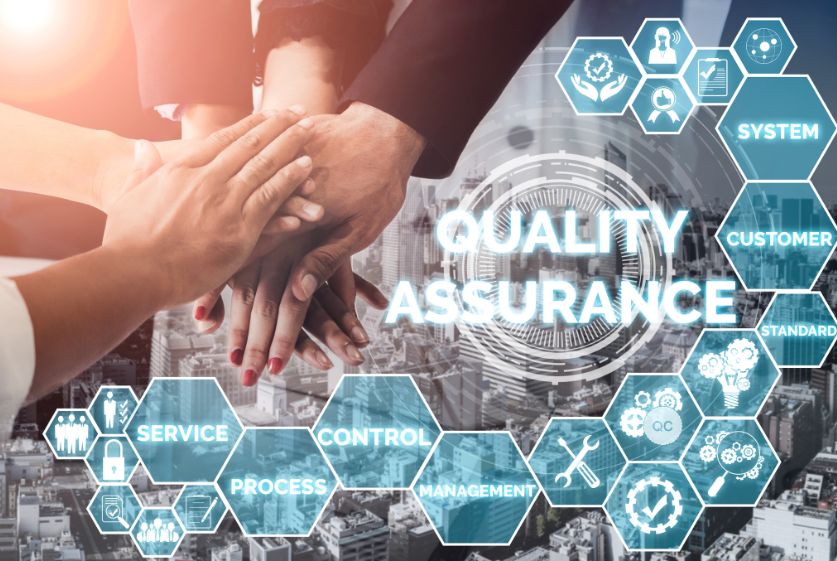
Complexity and Uncertainty
The real world is inherently complex and uncertain, presenting significant challenges for AI systems. Robust Artificial Intelligence must be capable of handling ambiguity, noise, and unexpected situations while still providing reliable and consistent outputs. This requires advanced techniques for modeling uncertainty, reasoning under uncertainty, and decision-making in complex environments.
Ethical Considerations
As AI systems become more prevalent and influential, ethical considerations must be at the forefront of their development. Robust Artificial Intelligence systems must be designed with transparency, accountability, and fairness in mind, ensuring that their decisions and actions are unbiased, explainable, and aligned with ethical principles.
Addressing ethical concerns is crucial for building trust and acceptance of AI technologies in society.
Approaches to Building Robust Artificial Intelligence Systems
Ensemble Methods
Ensemble methods involve combining multiple models or algorithms to improve overall performance and robustness. By leveraging the strengths of different models and mitigating their weaknesses, ensemble methods can reduce errors, increase accuracy, and enhance generalization capabilities. Techniques such as bagging, boosting, and stacking are commonly used in ensemble learning.
Adversarial Training
Adversarial training is a technique that exposes AI models to intentionally crafted adversarial examples during the training process. These adversarial examples are designed to exploit vulnerabilities in the model, forcing it to learn robust representations and improve its resilience against adversarial attacks. By incorporating adversarial training, AI systems can become more secure and reliable in real-world deployment scenarios.
Reinforcement Learning
Reinforcement learning (RL) is a powerful approach for building robust Artificial Intelligence systems that can learn and adapt through interaction with their environment. In RL, an agent learns to make optimal decisions by receiving rewards or penalties based on its actions and the resulting outcomes. This iterative process allows the agent to explore different strategies and refine its behavior, leading to robust and adaptive decision-making capabilities.
Real-World Applications of Robust Artificial Intelligence
Healthcare and Medical Diagnosis
Robust AI has the potential to revolutionize the healthcare industry by providing accurate and reliable medical diagnoses, even in the face of incomplete or noisy data.
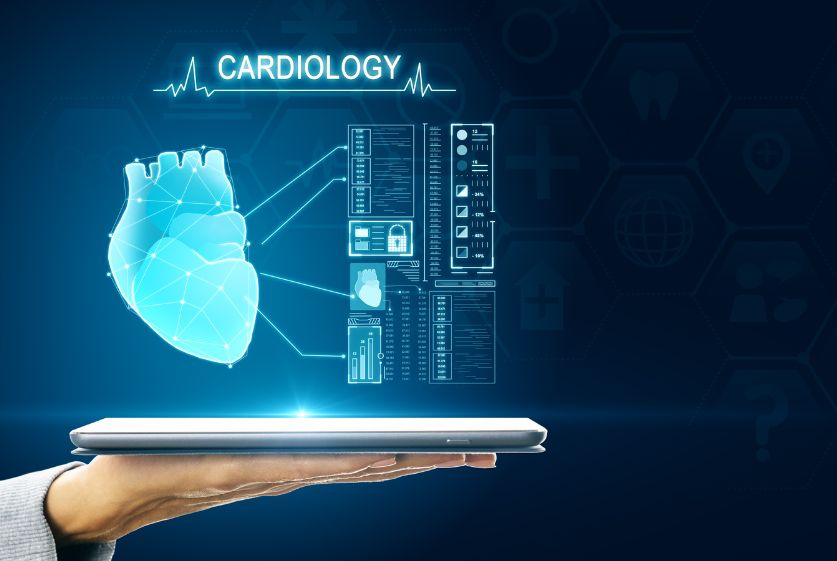
Robust Artificial Intelligence systems can analyze medical images, patient records, and clinical data to detect patterns and identify potential health issues while accounting for uncertainties and variations in data quality. This can lead to earlier diagnoses, personalized treatment plans, and improved patient outcomes.
Autonomous Vehicles and Transportation
The development of autonomous vehicles heavily relies on the robustness of AI systems. These vehicles must be capable of navigating complex environments, adapting to changing conditions, and making split-second decisions while ensuring the safety of passengers and other road users. Robust Artificial Intelligence algorithms are essential for perception, decision-making, and control in autonomous vehicles, accounting for unpredictable situations, sensor failures, and adversarial attacks.
Cybersecurity and Fraud Detection
In the realm of cybersecurity and fraud detection, Robust Artificial Intelligence can play a crucial role in identifying and mitigating threats. By analyzing network traffic, user behavior, and transaction data, robust AI systems can detect anomalies, recognize patterns, and adapt to emerging threats.
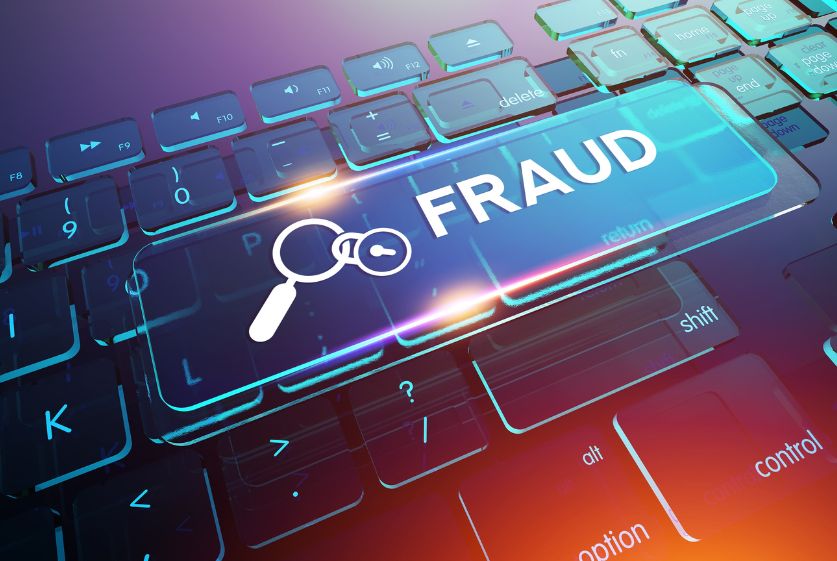
These systems must be resilient against adversarial attacks and capable of handling evolving tactics used by cybercriminals, ensuring the protection of sensitive data and financial assets.
The Future of Robust Artificial Intelligence
Emerging Trends and Advancements
The field of robust AI is rapidly evolving, with continuous research and innovation driving new advancements.
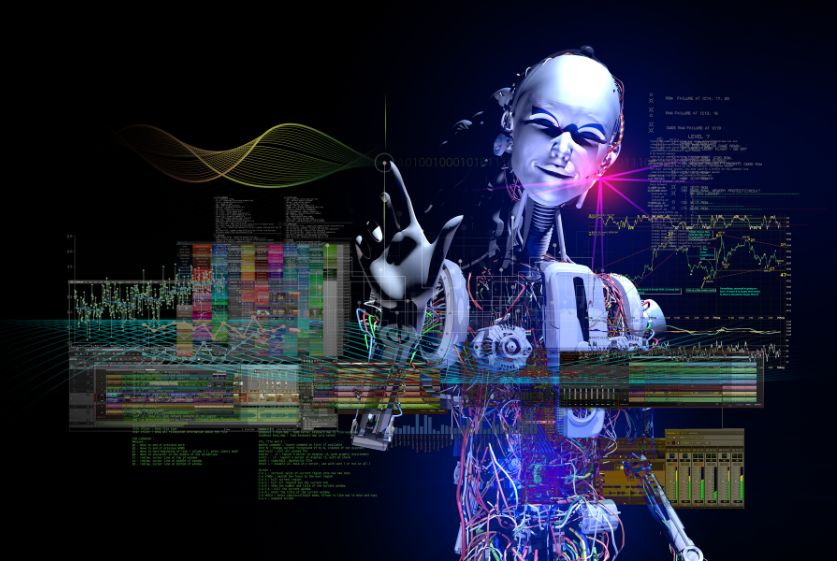
Some emerging trends and advancements include federated learning (which enables collaborative model training while preserving privacy), causal reasoning (for better understanding and modeling of cause-and-effect relationships), and the integration of AI with other technologies such as quantum computing and neuromorphic hardware.
Potential Impact on Industries and Society
As Robust Artificial Intelligence systems become more prevalent, their impact on various industries and society as a whole is expected to be significant. From optimizing supply chains and improving manufacturing processes to enhancing environmental monitoring and disaster response, robust AI has the potential to drive efficiency, reduce costs, and improve decision-making across multiple sectors.
The ethical and responsible development of robust AI will be crucial in ensuring its positive impact on society and addressing concerns related to privacy, fairness, and accountability.
Conclusion
The pursuit of robust artificial intelligence is a critical endeavor in the field of AI, as it addresses the need for reliable, adaptable, and trustworthy systems capable of operating in complex and uncertain environments. By incorporating key characteristics such as adaptability, fault tolerance, and scalability, robust AI systems have the potential to tackle a wide range of real-world challenges, from healthcare and autonomous vehicles to cybersecurity and fraud detection.
The development of robust AI is not without its challenges, including data quality issues, complexity, and ethical considerations. Overcoming these challenges will require innovative approaches, such as ensemble methods, adversarial training, and reinforcement learning, as well as a strong commitment to ethical and responsible AI development.
As research and development in robust AI continue to advance, the impact on industries and society is expected to be profound. From optimizing processes and improving decision-making to driving innovation and addressing global challenges, robust AI holds immense potential for shaping a better future.
The pursuit of robust AI is not just a technological endeavor but a testament to humanity’s resilience, adaptability, and commitment to creating intelligent systems that are trustworthy, reliable, and capable of enhancing our lives in meaningful ways.
FAQs
What is the difference between traditional AI and robust AI?
Traditional AI systems are often designed for specific tasks or environments, while robust AI systems are built to be resilient, adaptable, and reliable across a wide range of conditions and scenarios. Robust AI systems incorporate techniques to handle uncertainties, adversarial attacks, and changing environments.
Why is robustness important in AI systems?
Robustness is crucial for AI systems to be reliable and trustworthy in real-world applications. As AI becomes more integrated into critical infrastructure and decision-making processes, ensuring its robustness is paramount to minimize risks and maximize benefits. Robust AI systems can maintain high performance and reliability even in the face of uncertainties or adversarial attacks.
What are some common approaches to building robust AI systems?
Some common approaches to building robust AI systems include ensemble methods (combining multiple models), adversarial training (exposing models to adversarial examples), and reinforcement learning (learning through interaction with the environment). These techniques aim to improve the adaptability, fault tolerance, and generalization capabilities of AI systems.
How can robust AI impact industries and society?
Robust AI has the potential to drive efficiency, reduce costs, and improve decision-making across various industries, such as healthcare, transportation, cybersecurity, and manufacturing. It can also contribute to addressing global challenges, optimizing processes, and enhancing environmental monitoring and disaster response. However, the ethical and responsible development of robust AI is crucial to ensure its positive impact on society.
What are some ethical considerations in the development of robust AI?
Ethical considerations in the development of robust AI include transparency, accountability, fairness, and alignment with ethical principles. Robust AI systems must be designed to be unbiased, explainable, and respectful of privacy and individual rights. Addressing ethical concerns is crucial for building trust and acceptance of AI technologies in society.