Table of Contents
Quantum computing is an exciting new paradigm of computing that harnesses quantum mechanical phenomena like superposition and entanglement to achieve significant speedups for certain types of calculations. When paired with artificial intelligence (AI), quantum computing has the potential to drastically accelerate machine learning and enable new capabilities that push AI to the next level.
In this article, we’ll provide background on quantum computing and AI, discuss how will quantum computing affect Artificial Intelligence applications, highlight some concerns and challenges, and summarize the outlook for this powerful combination of technologies.
Background on Quantum Computing
To understand how quantum computing will influence AI, let’s first review some key principles behind this novel computing approach:
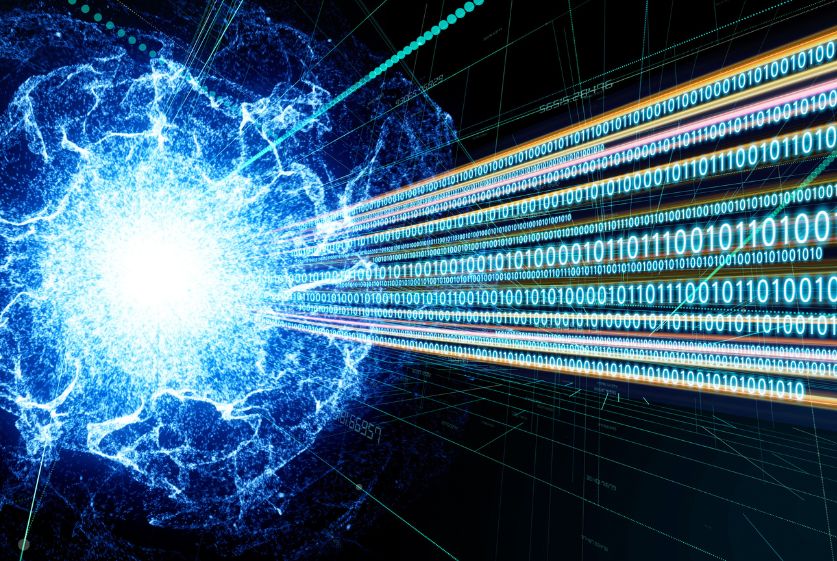
Qubits and Superposition
Unlike classical binary bits that can only be in a 0 or 1 state, quantum bits (qubits) can exist in a superposition of both states simultaneously. This allows qubits to represent and consider multiple combinations of values at once.
Quantum Parallelism
Related to superposition, quantum parallelism allows operations to be performed on all possible states of qubits simultaneously. This massively parallel processing capability is what fuels the potential speedups from quantum computers.
Quantum Algorithms
Special quantum algorithms like Shor’s algorithm for factoring integers and Grover’s algorithm for search tasks have been designed to take advantage of quantum mechanical effects for monumental efficiency gains not achievable by classical algorithms alone.
Background on Artificial Intelligence
Now let’s provide some context on AI:
Machine Learning
Machine learning involves developing algorithms that can learn patterns from data to make decisions or predictions without explicit programming. Key techniques like supervised, unsupervised, and reinforcement learning power many AI applications today.
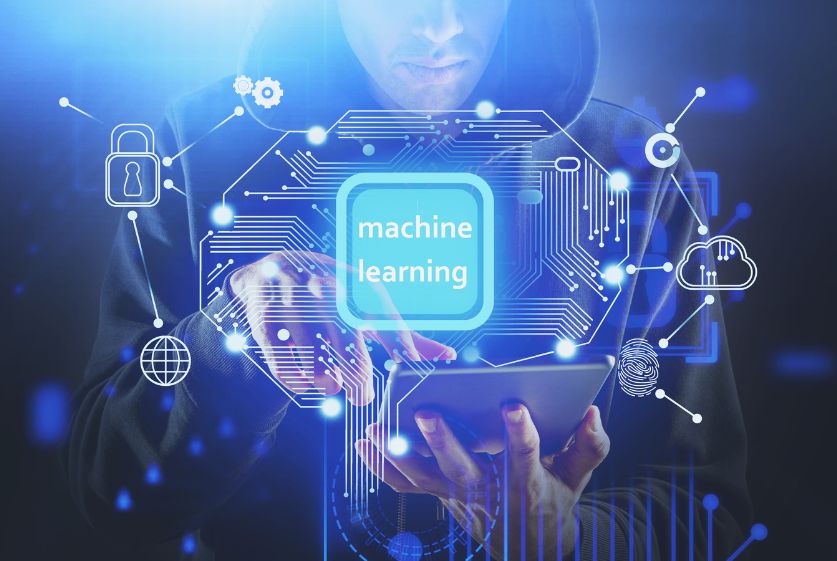
Neural Networks
Neural networks inspired by the human brain process and interpret data through interconnected nodes laid out in input, hidden, and output layers. By adjusting connection strengths during training, neural nets can recognize complex patterns for computer vision, speech recognition, and more.
Deep Learning
Deep learning uses large-scale neural nets with many hidden layers, leveraging massive amounts of data and computing power to achieve remarkable accuracy on complex tasks like image, video, and audio analysis.
How Will Quantum Computing Affect Artificial Intelligence Applications
With backgrounds in both fields covered, we can now dive into the main ways quantum computing will shape AI:
Faster Training of AI Models
One of the biggest potential impacts is significantly faster training times for machine learning and neural network models. Reasons this speedup is possible:
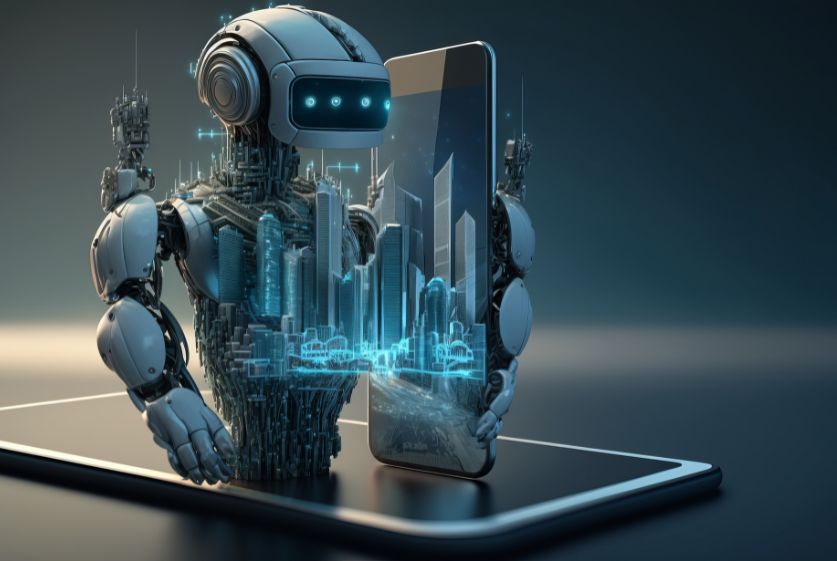
Complex Datasets
Quantum computers can rapidly process larger, more complex datasets to feed into training algorithms.
Parameter Tuning
The trial-and-error process of tuning model hyperparameters can be accelerated with quantum optimization algorithms.
Model Evaluation
Quickly evaluating model performance on validation datasets allows more rapid iterations during development. Together, these improvements could slash training times for advanced AI models from months down to days or even hours.
More Complex AI Models
In addition to faster training, quantum computing will enable more sophisticated AI models:
Deeper Neural Networks
Quantum tensor networks could simulate extremely deep neural networks with exponentially more parameters.

Larger Scale Problems
Issues currently out of reach like climate change modeling and cellular-level biological simulations could become feasible training tasks.
Hierarchical Learning
Hybrid classical quantum systems may allow complex hierarchical learning across nested subtasks. These possibilities will push AI capabilities to new frontiers.
New Capabilities
Furthermore, quantum mechanics could introduce entirely novel functionalities:
Quantum Machine Learning
Native quantum algorithms tailored for machine learning could offer exponential speedups compared to classical counterparts.
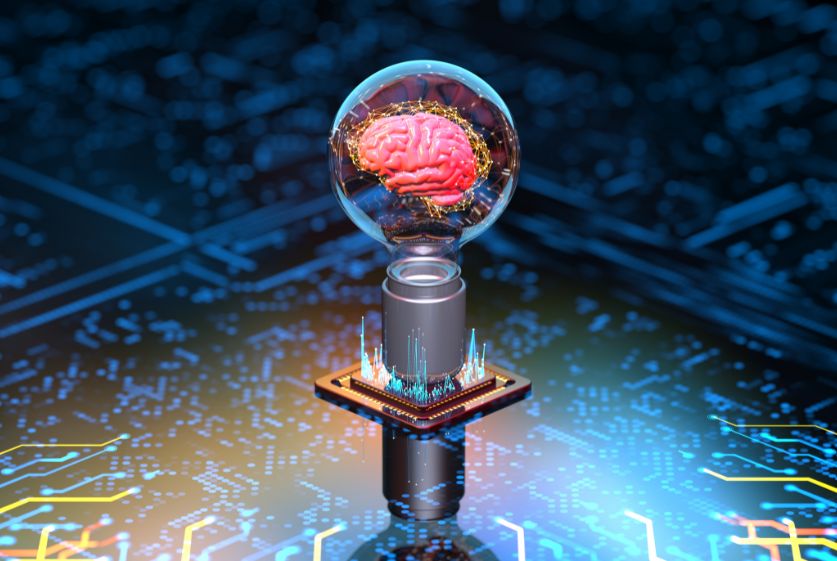
Quantum Neural Networks
Quantum neural network prototypes leverage superposition and entanglement for pattern recognition inside quantum computers.
Quantum Reinforcement Learning
Quantum versions of popular reinforcement learning models like Q-learning could discover smarter behavioral policies faster. Such new directions will expand the toolkit available to AI developers.
Challenges and Concerns
There are significant obstacles to address before quantum AI comes to full fruition:
Implementation Difficulties
Making quantum hardware reliably generate quantum effects poses physics and engineering hurdles:
Stable Qubits
The delicate superposition states used by qubits decay very rapidly, requiring sophisticated systems to maintain coherence.
Scaling Systems
Expanding from prototypes to the millions of qubits likely needed will demand new architectures and materials. Surmounting these issues demands continued research breakthroughs.
Security Vulnerabilities
Quantum technologies also introduce cybersecurity complications:
Encryption
Quantum computers threaten to crack today’s public key encryption schemes, requiring upgraded cryptographic standards to stay safe.
Algorithm Vulnerabilities
Adversaries could potentially spy on or corrupt quantum AI through subtle side channels during algorithm execution. Guarding against these hazards is critical as systems are built out.
Ethical Considerations
Additionally, thoughtfully addressing deeper societal impacts is vital:
Job Automation
The economic disruption from automating roles through advanced AI must be carefully managed at a policy level.
Bias and Fairness
Proactive safeguards need to combat potentially biased or unfair quantum AI systems that could negatively affect minorities.
Transparency
Explaining what drives quantum AI behavior will be challenging but important for human trust and oversight. Prioritizing ethics and safety should be paramount as exploration continues.
Future Outlook
The next decade promises great leaps toward usable quantum computers and increasingly powerful AI. With diligence and responsibility guiding progress, quantum-enhanced AI could unlock incredible breakthroughs and positively revolutionize many spheres of human life in the years to come.
Conclusion
The combination of quantum computing and AI holds enormous transformative potential alongside risks that must be diligently addressed.
Quantum physics promises faster training, more capable models, and entirely new functionalities to strengthen AI’s position as a dominant force in technology. However, realization depends on surmounting hardware implementation barriers while ensuring security and ethical soundness.
FAQs
Q: How much faster could AI training be with quantum computers?
A: Estimates suggest quantum computers could offer over 100 million times faster AI model training compared to classical systems – reducing long development cycles down to minutes.
Q: When will advanced quantum AI become a reality?
A: While limited proof-of-concept experiments exist today, most experts predict robust quantum AI applications are still 10-20 years away as hardware scales up and algorithms mature.
Q: What are the most promising areas for quantum AI?
A: Drug discovery, financial modeling, logistics optimization, climate forecasting, and cybersecurity are top domains expected to benefit tremendously from quantum AI augmentation.
Q: Is there a risk quantum AI could become too uncontrollable?
A: Yes, which is why researchers stress that safety and ethics need to be priority considerations at every step along the development journey to avoid harmful incidents.
Q: Who are the top tech companies investing in quantum AI?
A: Google, Microsoft, IBM, and Amazon all have major quantum computing groups working to accelerate quantum AI – a trend likely to expand industry-wide given the tremendous upside potential.