Table of Contents
Introduction to AI
Artificial intelligence (AI) refers to computer systems that can perform difficult tasks that typically require human intelligence. There are several types of AI, with two major categories being generative AI vs predictive AI. While both involve “smart” computer systems, they have some key differences in their purpose, approach, strengths, and limitations.
What is Generative AI?
Generative AI focuses on creating new, original content. The goal is for AI systems to generate products like text, images, video, and audio that humans would consider to be high-quality and valuable contributions.
Prominent examples of generative AI include systems like DALL-E for image generation and GPT-3 for text generation. These models can produce extremely realistic synthetic content.
What is Predictive AI?
Alternatively, predictive AI analyzes existing data and patterns to forecast future outcomes, trends, or behaviors. These systems identify potentially useful insights and make data-driven recommendations or decisions, but do not create anything new.
Common predictive AI applications include sales forecasting, risk assessment, predictive maintenance, and targeted advertising.
Key Differences Between Generative AI vs Predictive AI
While both involve AI systems, generative AI and predictive AI have significant differences that impact their use cases and performance.
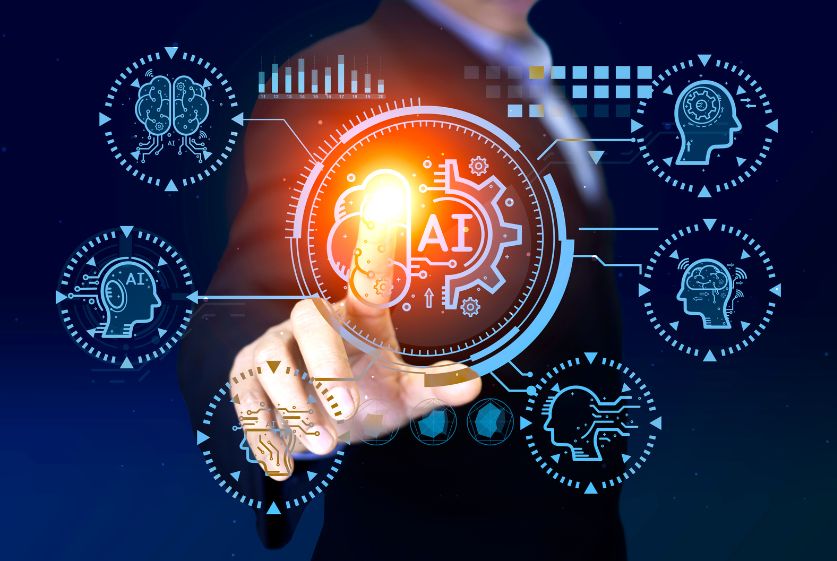
Purpose and Functionality
The core purpose varies significantly – generative AI produces new content while predictive AI analyzes data to predict future outcomes. They require fundamentally different functionalities to achieve these distinct goals.
Data Requirements
The data needs also differ. Generative AI models are trained on vast datasets of high-quality examples like text, images, and audio. Predictive AI may utilize a wider range of real-world data including numbers, categories, sensor readings, text, etc.
Model Training Approaches
The training processes have little in common. Generative models use unsupervised and self-supervised learning to uncover complex patterns and recreate content. Predictive models are mostly trained via supervised learning based on historical examples of inputs and desired outputs.
Performance Evaluation
The evaluation metrics are completely different. Generative AI quality focuses heavily on realism, coherence, creativity, and the ability to fool humans. Predictive AI centers on numerical performance metrics like accuracy, precision, recall, and F1 scores.
Unique Strengths of Each Type of AI
While they have significant differences, generative and predictive AI offer unique value in their own right.
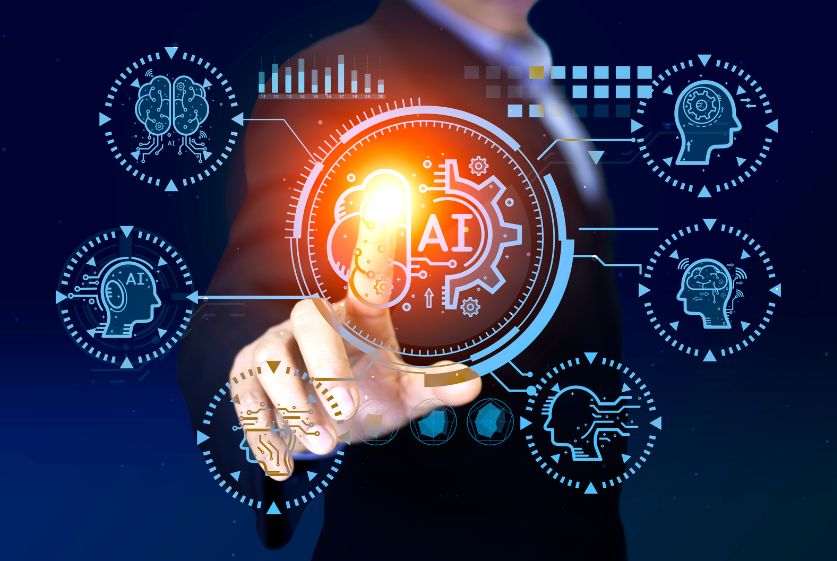
Generative AI Strengths
Generative AI offers exceptional creativity and content-generation abilities. Models like DALL-E 2 and GPT-3 display impressive capacities for generating realistic, high-quality synthetic content. The ability to automate creative tasks provides tremendous value.
Generative models can also personalize content and experiences in a customized, engaging way not manually at scale. They open possibilities for completely new types of content and interfaces.
Predictive AI Strengths
Predictive analytics powers data-driven decision-making through its ability to uncover insights and trends from data. Models provide data-backed recommendations and forecasts to optimize outcomes.
Predictive AI also enhances efficiency through its capabilities for process automation, predictive maintenance, and more. By understanding future needs and events, organizations can allocate resources appropriately.
Predictive AI supports consistency and standardization in forecasting, risk analysis, targeting, and other applications based on codified models rather than individual human whims.
Limitations of Generative and Predictive AI
Both varieties of AI also face notable limitations.
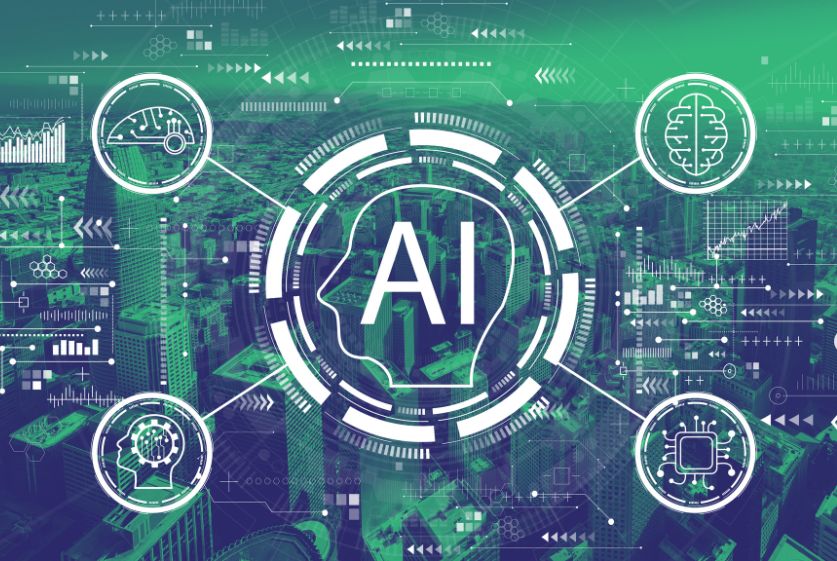
Generative AI Limitations
Despite fast-paced innovations, generative AI still faces challenges with logical consistency, factual accuracy, and responsible content generation. Without the contextual grasp of meaning and ethics that humans intrinsically possess, malicious or biased content remains a concern requiring ongoing governance.
Current generative models also lack troubleshooting capacities to resolve errors or biases on their own. The outputs are only as good as the data, parameters, and guidelines provided during the training process. Achieving models with more generalized critical thinking abilities remains an area for further research.
Predictive AI Limitations
Predictive AI relies fully on the quality of input data, meaning that incomplete data or hidden variables can undermine recommendations. Models may also propagate existing biases within historical data.
Interpretability remains an issue. The inner workings of complex predictive models are often opaque and difficult to explain, making it hard to troubleshoot errors or understand failures.
Finally, predictive AI cannot anticipate unexpected events like COVID-19’s impact on supply chains and purchasing patterns. Models based on historical data have limited capacity to account for unprecedented influences or scenario changes.
Real-World Applications
Both forms of AI present promising opportunities for real-world impact.
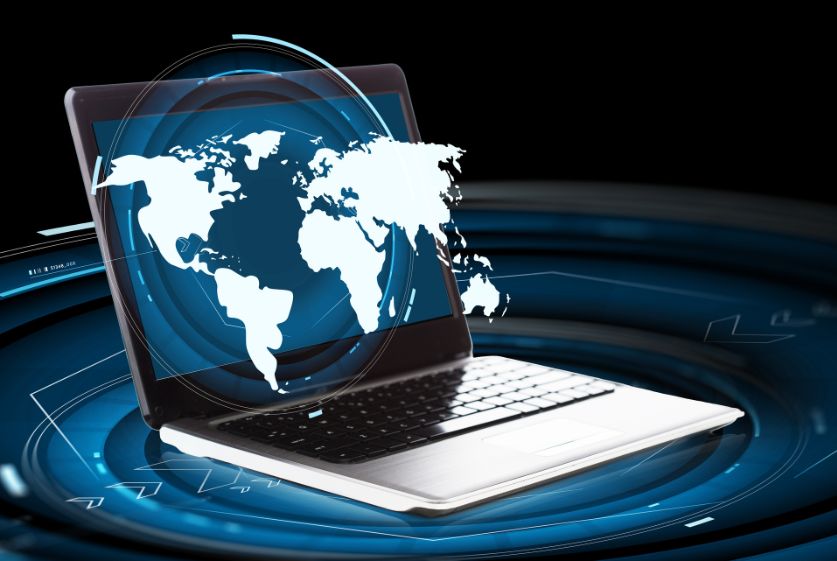
Generative AI Applications
Creative fields are already embracing AI generation for content creation, personalization, and enhancing human capabilities. Use cases include:
- Automatically generating articles, stories, dialogue, code, and more
- Personalizing marketing content for customized relevance
- Graphic design acceleration through AI-generated images, 3D models, and video
- Conversational interfaces and chatbots with natural dialogue
Generative AI also holds promise for drug discovery, materials science, cybersecurity enhancement, and accessibility via capabilities like real-time voice transcription.
Predictive AI Applications
Meanwhile, predictive analytics is powering data-driven innovation across:
- Marketing optimizations like predictive lead scoring and conversion rate modeling
- Financial forecasting for volatility modeling, fraud detection, and risk analytics
- Healthcare AI for optimized patient treatment plans and readmissions forecasting
- Predictive maintenance to maximize equipment uptime and optimize repair planning
- Supply chain analytics for inventory and shipment forecasting, dynamic pricing
The common theme is leveraging data to optimize outcomes.
The Future of Artificial Intelligence
Both major forms of AI have promising futures as research and development accelerates.
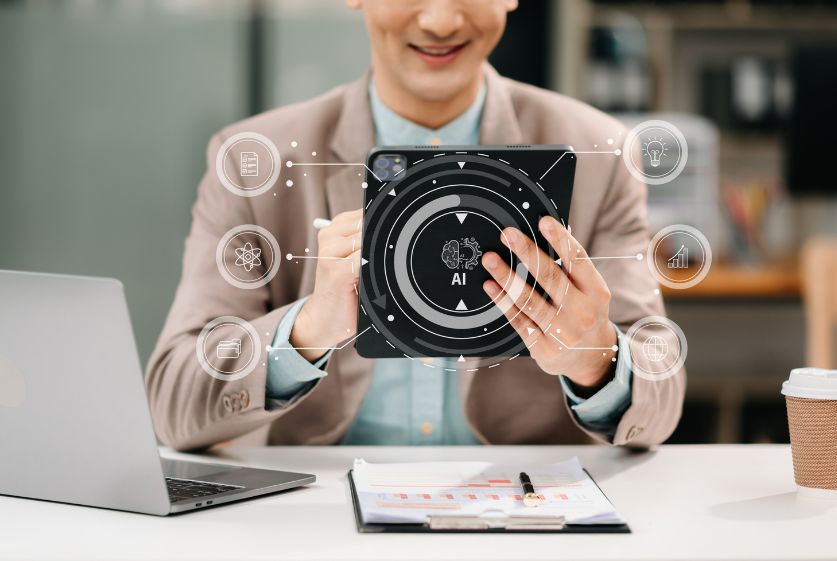
Advances in Generative AI
Generative models are rapidly evolving toward deeper contextual understanding, reasoning capacities, factual consistency, and responsible content production. Democratizing creativity holds transformative potential.
Advances in Predictive AI
Predictive analytics is also becoming more powerful and specialized for particular domains like healthcare, marketing, finance, and more. Improving data quality, transparency, and trust in automated decision-making remains a priority.
Complementary Systems
Generative and predictive AI offer complementary strengths to enhance human capabilities through task automation and intelligence augmentation. Powerful synergies may emerge from unifying advances across both realms of research over the coming years at the frontiers of artificial general intelligence.
Conclusion
Generative AI focuses on creating novel, original content while predictive AI analyzes data to forecast future outcomes. Key differences in their purpose, data requirements, model training process, and evaluation metrics lead to distinct strengths, limitations, and promising real-world applications.
Both offer tremendous value today and in the future, as AI capabilities continue rapidly evolving through innovations in models, data, and computing infrastructure. Though remaining fundamentally distinct branches of technology, synergies may form between advances in generative and predictive spheres.
FAQs
What are some common examples of generative vs predictive AI?
Generative AI creates original synthetic content like DALL-E AI art or GPT-3 essays. Predictive AI performs tasks like sales forecasting, predictive maintenance, or medical diagnosis using health data.
Is generative AI truly creative?
Today’s AI still has limitations in contextual reasoning, though models exhibit impressive capacities for generating creative, realistic content. Assessing if or when AI rivals human-level creativity remains an open debate.
Do generative and predictive models require different skills?
Yes. Generative AI places heavier emphasis on unsupervised learning, neural networks, data quality, and product evaluation. Predictive AI prioritizes skills like statistics, supervised learning, and industry-specific modeling.
Can one company utilize both generative and predictive AI?
Absolutely. For instance, an e-commerce site could leverage predictive analytics to optimize sales while using generative AI to automatically generate tailored product descriptions for customers. The synergistic applications are immense.
Which type of AI represents the future?
Both realms of AI innovation hold incredible promise and are rapidly evolving. Predictive and generative systems already deliver immense value across industries. The future likely involves both complementary varieties of AI advancing hand-in-hand.