Artificial intelligence (AI) systems rely heavily on data to function, learn, and improve – but without proper safeguards in place, the data powering AI can pose serious risks. As AI continues expanding into sensitive domains like healthcare, finance, employment, and more, protecting the data used to develop and operate AI systems is crucial.
Complete Artificial intelligence data protection entails privacy preservation, security defenses, reducing algorithmic bias, and implementing strong governance practices. As AI gains more influence globally, prioritizing robust and ethical data policies will help realize the vast benefits of the technology while building public trust.
Table of Contents
What is AI and How Does it Use Data?
AI Defined
Artificial intelligence refers to computer systems designed to perform tasks normally requiring human cognition and decision-making abilities. Key capabilities include learning, reasoning, problem-solving, perception, and even creativity.
Common AI examples include virtual assistants, self-driving vehicles, personalized recommendations, predictive analytics, and more.

At the core, AI systems are developed using data and algorithms. Programmers feed troves of data into machine learning algorithms, which reveal patterns within the data and use those patterns to make judgments and decisions about new data. As a result, the underlying data significantly impacts system development and performance.
Data Usage in AI Systems
The data used in AI powers every stage of the machine learning pipeline, including:
- Training data: Large datasets for building and testing algorithms
- Input data: Real-time data inputs AI uses for decision-making
- Performance data: Ongoing data to evaluate AI fairness, accuracy and reliability
Having abundant, high-quality data is key for AI functionality. The collection, storage, and usage of data also create opportunities for abuse if not handled carefully.
Risks and Challenges of Unprotected AI Data
AI data risks typically stem from improper or non-consensual data collection practices, security vulnerabilities enabling data breaches, or bias in algorithm design. Without focused efforts to protect data and govern usage responsibly, AI systems can seriously undermine people’s rights and well-being.
Privacy Issues
The mass collection of personal data for AI – including names, locations, browsing history, purchases, biometric details, and more – raises legitimate privacy concerns over how much data is needed, how it’s managed long-term, and informed consent protocols.
Failure to implement reasonable privacy safeguards around AI data heightens the risk of emotional, financial, or physical harm.
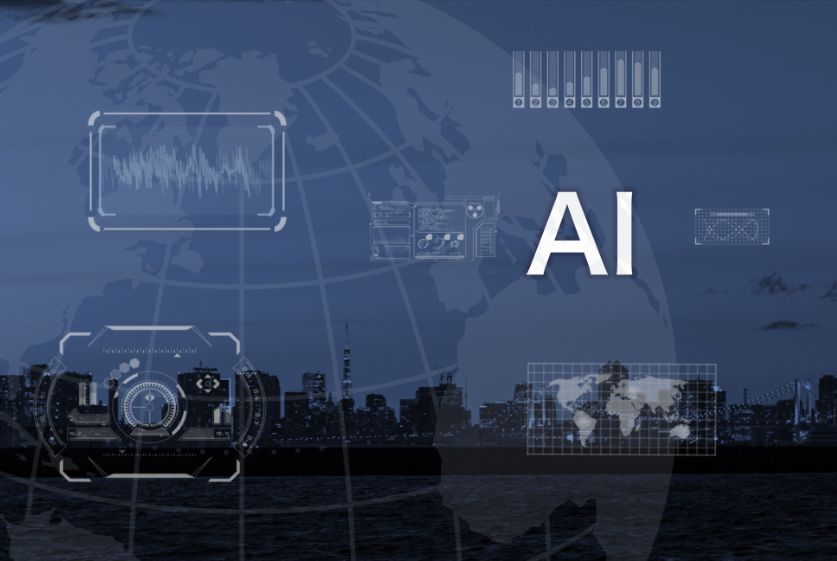
Security Threats
Like any valuable digital asset, AI data faces security threats from malicious hacking, theft, ransomware, and insider risks. Attackers could steal confidential data to enable identity fraud, compromise AI integrity through data poisoning, or disrupt operations by denying access. Proactive cybersecurity measures are essential.
Algorithmic Bias
Since AI algorithms inherit the patterns and biases found in data, mistakes or prejudice in dataset design can lead to unfair, unethical, or dangerous AI behavior.
Issues include under-representation, measuring the wrong metrics, or encoding human biases. Ongoing bias testing using diverse data helps increase AI reliability and fairness.
Strategies for Artificial Intelligence Data Protection
Protecting AI data requires a multi-layered approach addressing privacy, security, bias, and governance. Leading strategies include:
Access Controls
Managing authorized user access, multi-factor authentication, least privilege permissions, and careful monitoring through access logs reduce insider and external threats. Compartmentalizing sensitive personal data also limits exposure.
Encryption
Encrypting data in transit and at rest protects confidentiality by encoding information to render it unintelligible without keys. It’s a crucial safeguard against data theft.
Anonymization and Pseudonymization
Scrubbing datasets of directly identifiable information or using pseudonyms helps address many privacy risks in analytics while preserving data utility.
Testing for Bias
Continuously testing AI systems with diverse input data reflects inclusiveness, ensures sensitivity to all user groups, and meets ethical obligations around fairness and representation.
Developing Robust AI Data Governance
Beyond security controls and testing, organizations must implement responsible data policies and processes governing AI lifecycles.
Accountability and Transparency
Documenting data provenance, usage justifications, risk assessments, and mitigations increases ethical accountability and operational transparency.
Regulatory Compliance
Keeping up with evolving regulations around AI ethics and data usage demonstrates a commitment to people’s rights. Important laws include GDPR, algorithmic accountability acts, consumer privacy regulations, and non-discrimination mandates.
Continuous Monitoring
Assessing access patterns, unauthorized querying, model degradation and algorithmic bias over time through AI Ops solutions enhances risk visibility and system quality.
Future of Artificial Intelligence Data Protection
While ample challenges remain around AI data, focused governance, and technological innovation provide optimism for the future.
Emerging Technologies
Automating privacy enhancement, bias detection, data security, and compliance processes will ease protection demands on practitioners. Cryptographic techniques like homomorphic encryption and secure multi-party computation allow valuable data analysis without exposure.
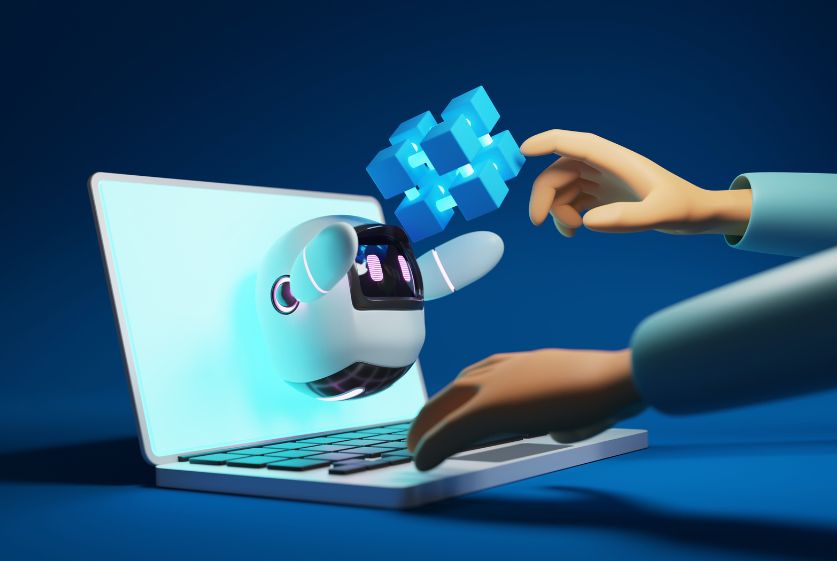
Public Discourse on Ethics
Elevating public discourse on AI ethics through education, opinion sharing, and policy debates will raise awareness of risks and encourage shared responsibility between the public and private sectors.
Global Policy Considerations
International collaboration on AI safety standards and best practices balances innovation with people’s rights. The OECD Principles on AI and the Council of Europe’s standards exemplify early efforts.
Upholding public trust in AI requires protecting the data fueling its advancement at each step while granting people meaningful control over their digital lives. Prioritizing purposeful governance, human rights, and ethical data usage will allow artificially intelligent technology to enhance human potential for the betterment of all.
Conclusion
From risks around privacy, security, and fairness to the growing dependency on AI permeating sensitive domains, protecting the data flowing through AI systems ranks among the most pressing challenges of the technology age if we are to realize its benefits without undermining human autonomy or well-being.
Technical safeguards, responsible governance, public discourse, and global collaboration all have roles to play in moving Artificial Intelligence data protection forward responsibly. With vigilance and collective caretaking, a future awaits where data and algorithms promote empowerment over-exploitation.
FAQs
How does data bias contribute to problems in AI systems?
Bias in the data used to build AI algorithms leads to inherited prejudice, underrepresentation, and unfair outcomes that compromise reliability and trust. Common issues include sampling bias, evaluation bias, variability bias, reporting bias, and historical bias rooted in past discrimination.
What privacy rights apply to personal data used by AI systems?
Laws like GDPR and CCPA grant people rights around consent, data minimization, restricted use cases, transparency, access requests, corrections, opt-outs, and deletion. Respecting these rights builds ethical AI and trust.
What is differential privacy and how does it help protect AI training data?
Differential privacy adds mathematical noise to aggregate dataset analytics to prevent revealing individual data points. This allows organizations to glean useful AI insights from sensitive data without exposing identities.
How can blockchain technology improve AI data governance?
The immutable ledger, automation, and decentralization of blockchain platforms lend themselves to use cases like tamper-proofing data, enabling user consent preferences, documenting data lineage, and restricting access only to validated parties.
What security measures protect against threats to AI model integrity?
Controls like code repositories, model versioning, anomaly detection systems, containerization, encrypted communications between components, and multi-factor access authentication all help defend AI pipelines against data poisoning attacks or model theft.