In the era of big data, artificial intelligence advanced analytics have emerged as game-changing technologies, that transform the way we process, analyze, and derive insights from vast amounts of information. These powerful tools are transforming industries, driving innovation, and unlocking new opportunities for businesses and organizations alike.
As we navigate through an increasingly data-driven world, the ability to harness the power of AI and advanced analytics has become a crucial competitive advantage. From personalized recommendations and predictive maintenance to fraud detection and disease diagnosis, these technologies are reshaping virtually every aspect of our lives.
In this complete article, I will share my experience with the fascinating world of AI and advanced analytics, delving into their definitions, applications, and the synergistic relationship between them. We will also discuss practical strategies for implementing these technologies, address ethical considerations, and provide a glimpse into the future of this rapidly evolving field.
Table of Contents
Understanding Artificial Intelligence
What is Artificial Intelligence?
Artificial Intelligence (AI) is a branch of computer science that aims to create intelligent machines capable of performing tasks that typically require human intelligence, such as reasoning, learning, problem-solving, perception, and decision-making. AI systems are designed to mimic human cognitive functions and adapt to new situations and environments.
The idea of AI dates back to the 1950s when computer scientists and researchers began exploring the possibility of creating machines that could think and reason like humans. Over the years, AI has evolved into various forms, including narrow AI (focused on specific tasks), general AI (on par with human intelligence), and super AI (surpassing human intelligence).
Applications of AI
AI has found numerous applications across a wide range of industries, from healthcare and finance to transportation and entertainment. Some notable examples include:
- Virtual assistants (e.g., Siri, Alexa, Google Assistant)
- Recommendation systems (e.g., Netflix, Amazon)
- Fraud detection and prevention
- Autonomous vehicles
- Image and speech recognition
- Predictive maintenance and optimization
- Personalized medicine and drug discovery
The adoption of AI has brought significant benefits to organizations, such as increased efficiency, improved decision-making, and enhanced customer experiences.
Exploring Advanced Analytics
What are Advanced Analytics?
Advanced analytics refers to a set of techniques and tools used to extract valuable insights from complex data sets, enabling organizations to make data-driven decisions and gain a competitive edge. It goes beyond traditional descriptive and diagnostic analytics by incorporating predictive and prescriptive capabilities.
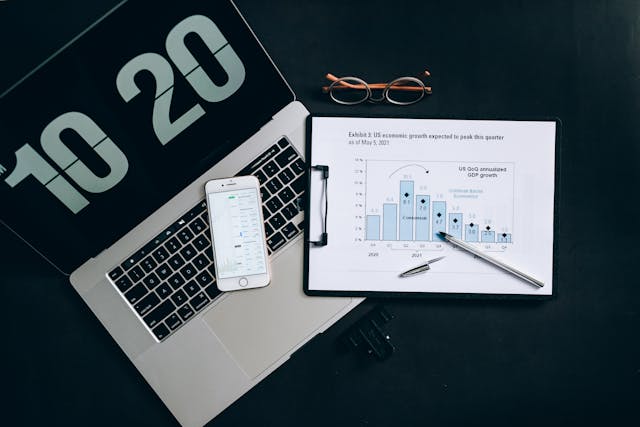
Advanced analytics leverages various methods, including machine learning, deep learning, natural language processing, and data mining, to uncover patterns, relationships, and trends that may not be readily apparent.
The Power of Advanced Analytics
Advanced analytics empowers organizations to:
- Predict future outcomes: By analyzing historical data and identifying patterns, advanced analytics can forecast future events, behaviors, or trends with a high degree of accuracy.
- Prescribe optimal solutions: Advanced analytics not only predicts potential outcomes but also provides recommendations and prescriptive actions to achieve desired results or mitigate risks.
- Drive data-driven decision-making: With the ability to process and analyze vast amounts of data, advanced analytics enables organizations to make informed, data-driven decisions, leading to better outcomes and improved competitiveness.
The Synergy of Artificial Intelligence Advanced Analytics
While AI and advanced analytics are distinct fields, they are highly complementary and often work in tandem to unlock even greater value from data. AI provides the computational power and algorithms necessary for advanced analytics, while advanced analytics techniques fuel AI systems with the insights and knowledge required for intelligent decision-making.
By combining the strengths of AI and advanced analytics, organizations can achieve:
- Automated and scalable data analysis: AI automates the process of data analysis, enabling organizations to process and analyze large volumes of data quickly and efficiently.
- Improved accuracy and personalization: Advanced analytics techniques, coupled with AI’s ability to learn and adapt, lead to more accurate predictions and personalized experiences.
- Real-time decision-making: The integration of AI and advanced analytics allows for real-time data processing and decision-making, enabling organizations to respond rapidly to changing conditions and opportunities.
Numerous industries have already leveraged the synergy of AI and advanced analytics to drive innovation and gain a competitive edge.
For example, in healthcare, AI-powered advanced analytics is used for early disease detection, personalized treatment plans, and drug discovery.
In finance, these technologies are employed for fraud detection, risk management, and investment portfolio optimization.
Implementing AI and Advanced Analytics
While the potential benefits of AI and advanced analytics are undeniable, successful implementation requires careful planning and execution.
Building the Right Team
Assembling a cross-functional team with diverse skills and expertise is crucial for effective AI and advanced analytics initiatives. This team should include:
- Data scientists: Responsible for developing and deploying AI and advanced analytics models, as well as interpreting and communicating findings.
- Data engineers: Responsible for collecting, cleaning, and managing large datasets to ensure data quality and accessibility.
- Subject matter experts: Domain experts who provide context and insights relevant to the specific business challenges or applications.
- IT professionals: Responsible for managing the underlying infrastructure, integrating systems, and ensuring data security and governance.
- Business leaders: Stakeholders who define the strategic goals, prioritize initiatives, and drive adoption within the organization.
Fostering collaboration and effective communication among these roles is essential for successful AI and advanced analytics projects.
Data Preparation and Management
The quality and reliability of data are critical for AI and advanced analytics initiatives. Organizations must establish robust data governance practices and invest in the following areas:
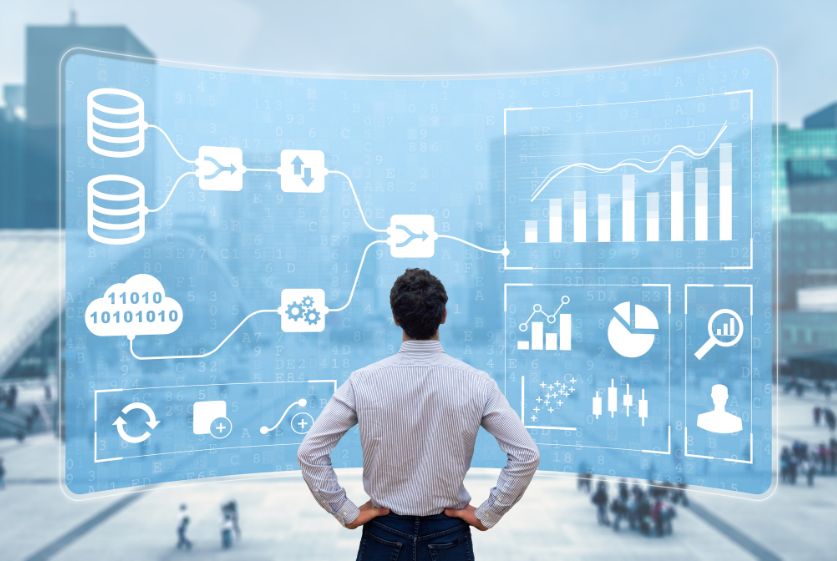
- Data collection and integration: Gathering data from various sources (internal and external) and integrating it into a centralized repository.
- Data cleaning and preprocessing: Ensuring data is accurate, consistent, and free from errors or inconsistencies.
- Feature engineering and selection: Identifying and extracting the most relevant features from the data to improve model performance and interpretability.
- Data security and privacy: Implementing measures to protect sensitive data and ensure compliance with relevant regulations (e.g., GDPR, HIPAA).
Effective data preparation and management lay the foundation for accurate and reliable AI and advanced analytics models.
Choosing the Right Tools and Technologies
The AI and advanced analytics landscape is constantly evolving, with new tools and technologies emerging regularly. Organizations must carefully evaluate their needs and select the appropriate solutions, considering factors such as scalability, performance, ease of use, and compatibility with existing systems.
Some popular AI and advanced analytics tools and platforms include:
- Machine learning frameworks: TensorFlow, PyTorch, sci-kit-learn (for Python), Microsoft Cognitive Toolkit (for C#), and others.
- Deep learning libraries: Keras, TensorFlow, Caffe, and PyTorch.
- Data visualization tools: Tableau, Power BI, and Qlik.
- Cloud platforms: Amazon Web Services (AWS), Microsoft Azure, and Google Cloud Platform.
Organizations may need to invest in hardware resources, such as high-performance computing (HPC) systems or graphics processing units (GPUs), to support resource-intensive AI and advanced analytics workloads.
Ethical Considerations
As AI and advanced analytics become more prevalent, it is crucial to address the ethical implications and ensure responsible development and deployment of these technologies.
Bias and Fairness in AI Systems
AI systems can inherit and amplify biases present in the training data or algorithms, leading to unfair and discriminatory outcomes. Organizations must take proactive measures to identify and mitigate potential biases, such as:
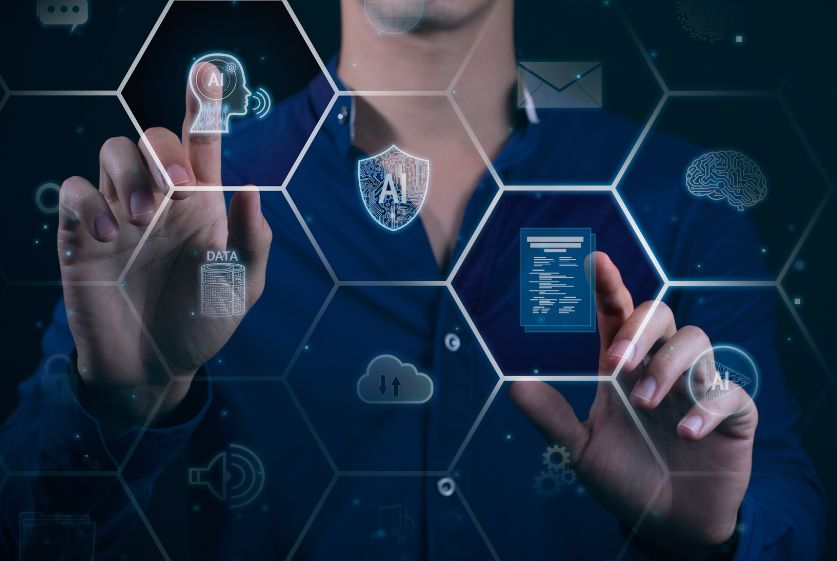
- Check diversity and representativeness in training data.
- Implementing techniques like adversarial debiasing and calibrated data augmentation.
- Conducting rigorous testing and auditing for biases.
- Promoting transparency and explainability in AI systems.
Privacy and Security Concerns
The vast amounts of data collected and processed by AI and advanced analytics systems raise privacy and security concerns. Organizations must prioritize data protection and implement robust security measures, such as:
- Anonymizing and encrypting sensitive data.
- Adhering to data privacy regulations (e.g., GDPR, CCPA).
- Employing secure data storage and transfer protocols.
- Implementing access controls and monitoring mechanisms.
- Conducting regular security audits and penetration testing.
Responsible AI Development and Deployment
Beyond addressing bias and privacy concerns, organizations should adopt a holistic approach to responsible AI development and deployment. This includes:
- Establishing an ethical AI governance framework and guidelines.
- Engaging stakeholders and fostering public trust and acceptance.
- Ensuring transparency and interpretability of Artificial Intelligence systems.
- Continuously monitoring and auditing AI systems for unintended consequences.
- Promoting workforce education and training on AI ethics and responsible use.
By prioritizing ethical considerations, organizations can mitigate risks, build trust, and ensure the responsible and beneficial use of AI and advanced analytics technologies.
The Future of AI and Advanced Analytics
The field of AI and advanced analytics is fast evolving, with breakthroughs and innovations emerging constantly. Here are some exciting trends and developments to watch out for:
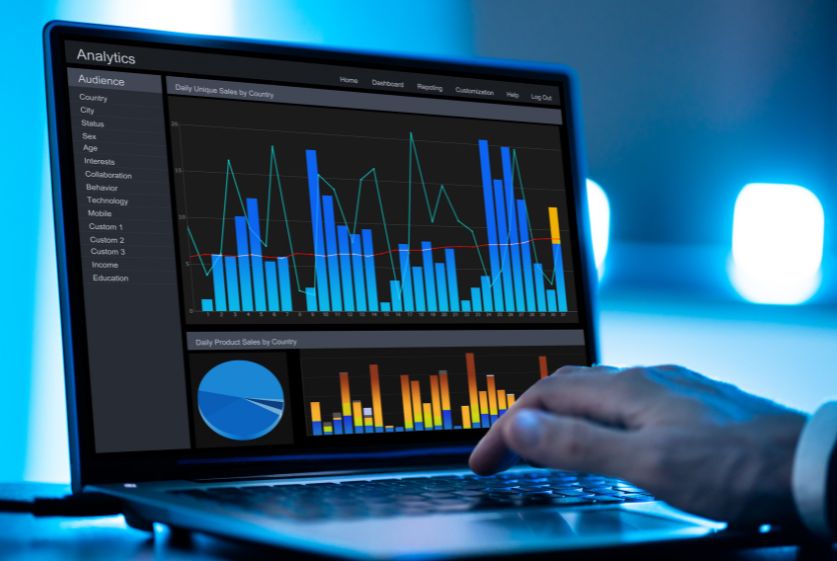
- Explainable AI (XAI): Efforts to make AI systems more transparent and interpretable, enabling humans to understand the reasoning behind AI-driven decisions.
- Federated learning and privacy-preserving analytics: Techniques that enable collaborative model training and analytics while preserving data privacy.
- Edge AI and Internet of Things (IoT): AI capabilities are being integrated into edge devices and IoT systems for real-time processing and decision-making.
- Generative AI: AI models capable of generating new content, such as text, images, audio, and video, with potential applications in creative industries.
- Quantum computing and AI: Leveraging quantum computing capabilities to accelerate AI training and tackle complex optimization problems.
As these advancements continue, AI and advanced analytics will likely permeate every aspect of our lives, transforming industries and shaping the future of work, healthcare, education, and beyond.
Conclusion
Artificial Intelligence and advanced analytics are powerful tools that are revolutionizing the way we process, analyze, and derive insights from data. By combining the computational power of AI with the predictive and prescriptive capabilities of advanced analytics, organizations can unlock new opportunities, drive innovation, and gain a competitive edge.
Successful implementation of AI and advanced analytics requires a strategic approach, involving the right team, robust data management practices, and carefully selected tools and technologies. Addressing ethical considerations, such as bias, privacy, and responsible development, is crucial to ensuring the beneficial and trustworthy use of these technologies.
As we look towards the future, AI and advanced analytics will continue to evolve, offering exciting new possibilities and shaping the way we live, work, and interact with the world around us. Organizations that embrace these technologies and adapt to the changing landscape will be well-positioned to thrive in the data-driven economy.
FAQs
What is the difference between AI and advanced analytics?
Artificial Intelligence (AI) refers to the development of intelligent machines capable of performing tasks that typically require human intelligence, such as reasoning, learning, problem-solving, and decision-making. Advanced analytics, on the other hand, is a set of techniques and tools used to extract valuable insights from complex data sets, enabling data-driven decision-making through predictive and prescriptive capabilities.
What are some real-world applications of AI and advanced analytics?
AI and advanced analytics have numerous applications across various industries, including healthcare (personalized medicine, drug discovery, early disease detection), finance (fraud detection, risk management, investment portfolio optimization), transportation (autonomous vehicles, predictive maintenance), retail (personalized recommendations, supply chain optimization), and many more.
How can organizations ensure ethical and responsible use of AI and advanced analytics?
Organizations should prioritize ethical considerations by addressing potential biases in AI systems, implementing robust data privacy and security measures, establishing an ethical AI governance framework, promoting transparency and interpretability, and continuously monitoring for unintended consequences. Workforce education and public engagement are crucial for fostering trust and acceptance.
What are some emerging trends in AI and advanced analytics?
Some exciting trends include explainable AI (XAI), federated learning and privacy-preserving analytics, edge AI and Internet of Things (IoT) integration, generative AI, and the application of quantum computing to accelerate AI training and optimization. Some exciting trends include explainable AI (XAI), federated learning and privacy-preserving analytics, edge AI and Internet of Things (IoT) integration, generative AI, and the application of quantum computing to accelerate AI training and optimization.
How can organizations prepare for the future of AI and advanced analytics?
To prepare for the future, organizations should stay informed about the latest developments and trends, invest in upskilling and reskilling their workforce, foster a culture of innovation and experimentation, and develop a strategic roadmap for AI and advanced analytics adoption. They should prioritize ethical and responsible practices, ensuring they are well-positioned to leverage these technologies for long-term success and competitiveness.